Analysing physical match performance
Which variables to look at?
The physical performance of football players is one of the contributing factors that has an impact on the match result. Obviously, the physical output of a player, such as the total distance covered, depends on a multitude of factors relating to playing style and match events. There are however several reasons why it is interesting to analyse the physical output of players.
By monitoring the output over multiple matches and season periods, patterns can be analysed to evaluate the physical development of players or to shed light on relevant deviations that may occur during the season.
For example, when a player underperforms relative to his historic data, an evaluation session can be organised to understand probable causes (e.g., in combination with video analysis) or to coach the player on aspects that need to be improved. Another reason to analyse the match output is the development of player-specific benchmarks that can be used to mimic match scenarios during training to improve performance.
These benchmarks can also be used as training targets for youth players during their development towards the first team (see one of our own articles published in Science and Medicine in Football).
How do we measure the physical output?
Since 2015, FIFA allows the use of electronic performance and tracking systems (EPTS) during official matches.
EPTS is an umbrella term for all systems that track players’ positions and movements on the field. It includes optical tracking systems, local positioning systems and global navigation satellite systems such as the global positioning system (GPS). These systems differ in their methodology but provide similar variables. For this blog, we will use GPS as an example system.
During matches, players wear GPS devices on the upper back of their body using specifically designed garments. This device receives low-powered radio signals from connected satellites at the velocity of light. Signals from a minimum of 4 satellites are required to find the exact location of the device, which is trigonometrically determined based on the signal transit time (i.e., time between arrival and transmission of the signal).
Based on the collection of positional data over time, variables related to the distance, velocity, and acceleration can be calculated using different methods such as positional differentiation or the Doppler-shift method.
These variables are often grouped in different bands to distinguish high-intensity from low-intensity movements, and to analyse both the distance, time and number of efforts within these bands. This results in a wealth of variables that can be used to analyse the match output. However, more data is not always better and makes the analysis certainly not easier or less complicated.
Therefore, a question often asked is:
Which external load variables do we have to look at?
Physical performance is multidimensional and is therefore best described by the interaction of multiple variables. However, because the description of multiple variables complicates the analysis, the visualisation and dissemination of data, practitioners are interested in reducing the dimensions of the dataset. They could select their key performance variables based on their preferences or their previous experiences, but a more valid approach would be to apply statistical dimension reduction techniques.
Such a technique is principal component analysis that can be applied to reduce the number of variables while not losing the veracity of information provided by these data. In this analysis, the physical output (also called the external load) is considered a latent variable which means that it cannot be directly observed but can be inferred from other variables that are observed such as the different GPS variables.
It has been shown that these variables are often highly correlated to each other. For example, when a player covers more distance in total, it can be expected that the distance at low speed or acceleration is also increased. The aim of principal component analysis is to create new uncorrelated variables that maximise the variance in the dataset describing the latent variable of interest (i.e., match output). These new variables (also called components) contain a set of the original variables that are correlated with each other, whilst the components themselves do not correlate.
Based on this analysis, one can use the new component scores to describe the physical output or one may select the variables that are most important for each component. Because most of the variance in the dataset can often be explained by 2 to 4 components, this analysis allows to reduce the variables from more than 50 to less than 5.
Different studies have applied principal component analysis to analyse the physical match performance. While we do not go into detail about these studies, the overall outcome of these studies is that the physical output is best described by a component of overall running load (e.g., total distance), a component of high-speed running load (e.g., high-speed running > 18 km.h-1), and a component of the acceleration and deceleration load (e.g., acceleration efforts > 2 m.s-2).
It may be useful to conduct a principal component analysis on own historical data but because such analyses are complicated, practitioners may trust the information delivered by previous studies. Therefore, we advise analysing the physical performance based on 3 variables that are related to these components. Of course, these variables will not always tell the full story but already aid in structuring the performance analysis.
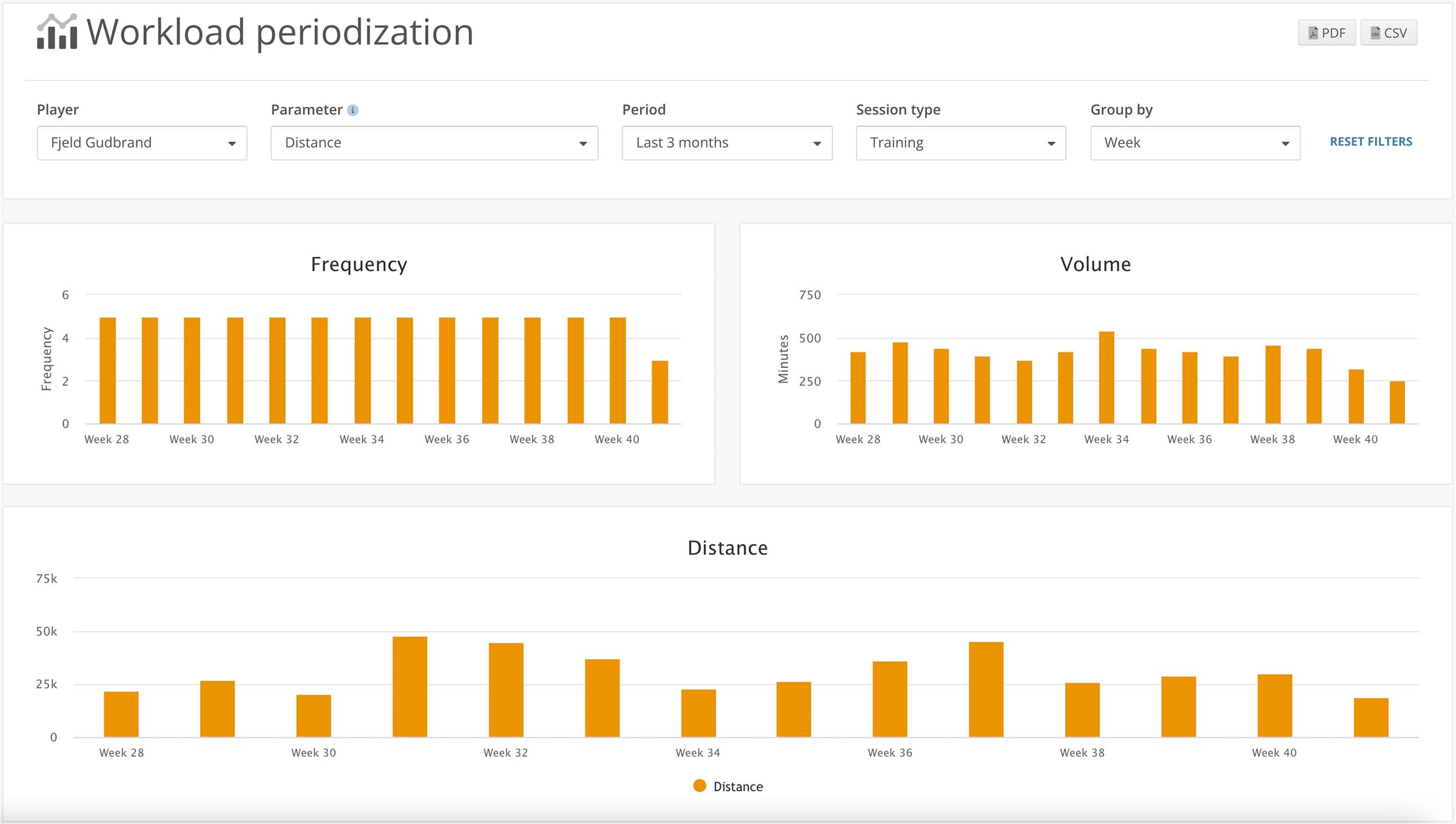
Interested in load monitoring?
Want to see how our platform can help you easily monitor training load with RPE?
Care to keep updated?
Subscribe to our newsletter.
You'll get updates about our recent blog posts and platform updates.
References
- Bradley, P. S., & Ade, J. D. (2018). Are current physical match performance metrics in elite soccer fit for purpose or is the adoption of an integrated approach needed? International Journal of Sports Physiology and Performance, 13(5), 656-664.
- Oliva-Lozano, J. M., & Muyor, J. M. (2021). Understanding the FIFA Quality Performance Reports for Electronic Performance and Tracking Systems: From Science to Practice. Science and Medicine in Football, (just-accepted).
- Ryan, S., Kempton, T., & Coutts, A. J. (2020). Data reduction approaches to athlete monitoring in professional Australian football. International Journal of Sports Physiology and Performance, 1(aop), 1-7.
- Maughan, P. C., MacFarlane, N. G., & Swinton, P. A. (2021). Relationship Between Subjective and External Training Load Variables in Youth Soccer Players. International journal of sports physiology and performance, 1(aop), 1-7.